AI for Wheat - Using Drones for Crop Height Estimation
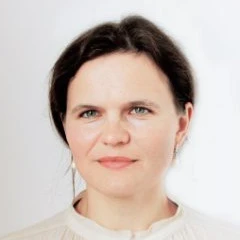
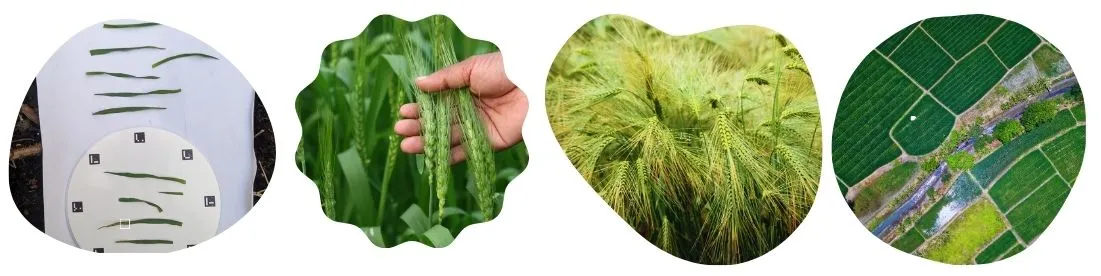
Why predicting wheat crop growth is important?
Predicting wheat crop growth, particularly crop height, is important because it serves as a vital indicator of overall plant health, directly influencing yield potential.
However, this method is historically approved, a farmer cannot accurately predict crop yield with just a ruler because yield prediction involves more than just measuring the height of the crops.
Measurement of crop height of wheat crop at agriculture farm, BHU campus, Varanasi. Source: Kumar et al., 2020
Accurate predictions of crop height allow farmers require more complex tools to assess how well their wheat is developing. Early identification of growth patterns through height predictions can also help detect issues like nutrient deficiencies, pest infestations, or diseases, allowing for quick interventions that prevent further damage.
By accurately predicting crop height, farmers can assess the likelihood of lodging and take preventive measures. For example, they can adjust planting density, apply growth regulators, or modify irrigation practices.
Examples of different plant height in wheat crops. Wheat: ‘Dagon’ (centre right) with reference varieties ‘Easton’ (centre left), ‘Sable’ (left) and ‘AAC Fairwind’ (right). Source: Canadian Food Inspection Agency
Important factors for crop height measurement
When checking crop height, here are the three most important things to keep in mind:
-
Growth Stage: Crops grow at different rates during their life, so it’s important to measure height at the same stage of growth each time to get accurate results.
-
Environmental Conditions: Factors like weather, soil, and watering can greatly affect how tall crops grow. It’s important to consider these conditions because they can change how the plants develop.
-
Accurate Measurements: Whether you’re measuring by hand or using tools like drones, it’s crucial to get accurate and consistent measurements. Good data helps you make the right decisions about how to care for your crops and avoid problems like plants falling over (lodging).
Phenotyping pipeline for estimation of plant height using UAV platform. DTM digital terrain model, DSM digital height model, PSM plant surface model, UAV unmanned aerial vehicle. Source: Hassan et al., 2019
Case Study on using machine learning for field-scale winter wheat growth prediction
Field-Scale Winter Wheat Growth Prediction Using Machine Learning**
Country: South Africa 🇿🇦
Published: 27 February 2024
This study explores how machine learning methods combined with unmanned aerial vehicle (UAV) imagery and soil properties can predict the growth of winter wheat at a field scale, aiming to enhance crop management and yield prediction.
Methodology flowchart for intra-field crop growth modelling used in this study. Nduku et al., 2024
The researchers utilized UAV-derived multispectral imagery to calculate vegetation indices (VIs) and gathered in situ soil property data.
Summary of analytical soil physical and chemical properties. Nduku et al., 2024
They applied four different machine learning regression models—Gaussian Process Regression (GPR), Ensemble Regression (ER), Decision Tree (DT), and Support Vector Machine (SVM)—to predict crop height at two wheat farms.
The study involved collecting UAV imagery at different growth stages and integrating this with soil data to improve prediction accuracy.
Map showing the location of the Clarence wheat farms in the Thabo Mofutsanyane district in the Free State province of South Africa. Nduku et al., 2024
Average monthly meteorological rainfall and temperature data from January to December 2021. Nduku et al., 2024
Multi-rotor DJI Matrice 600 Pro system equipped with MicaSense RedEdge-MX sensor (a) and a MicaSense Calibration Reflectance Panel serial number: RP04-1918107-OB (b). Nduku et al., 2024
Key findings revealed that the GPR model performed the best, with an R² of 0.69 to 0.74 and an RMSE of 15.95 to 17.91 cm, especially when using vegetation indices alone.
The RedEdge Normalized Difference Vegetation Index (RENDVI) was identified as the most influential predictor of wheat crop height. The study highlights that integrating UAV-based vegetation indices with soil data can significantly improve crop growth predictions, which is crucial for optimizing farm management practices.
Farm A spectral bands’ surface reflectance of wheat at different stages ((A) = Blue, (B) = Green, (C) = Red, (D) = RedEdge, (E) = NIR). The frequency values are scaled. Nduku et al., 2024
Farm B spectral bands’ surface reflectance of wheat growth canopies at different stages ((A) = Blue, (B) = Green, (C) = Red, (D) = RedEdge, (E) = NIR). The frequency values are scaled. Nduku et al., 2024
These findings can be practically applied by agricultural researchers, farm managers, and precision agriculture practitioners to improve crop monitoring and yield prediction using cost-effective UAV and AI technologies.
Main Tools / Technologies Used:
- UAV with MicaSense RedEdge-MX sensor
- Gaussian Process Regression (GPR)
- Ensemble Regression (ER)
- Decision Tree (DT)
- Support Vector Machine (SVM)
- Vegetation indices (RENDVI, NDVI, NDI, RVI2)
- MATLAB software for data processing and modeling
Reference: Nduku et al., 2024
Key Takeaways for Designing Experiments on Wheat Crops
The methodology for this research on wheat crop height prediction has several distinctive aspects which might be helpful for your work:
-
Combination of UAV Imagery and Machine Learning. Combining UAV imagery with machine learning offers precise crop height predictions. You can use this method for monitoring other crops where growth patterns are critical.
-
Field-Scale Application. Applying predictive models at the field level is useful for large-scale farming operations aiming to optimize crop management.
-
Soil Data Incorporation. Including soil measurements enhances prediction accuracy. Consider using this method for crops where soil conditions significantly impact growth and the data is available.