AI for Potato - USDA Grading with mobile device
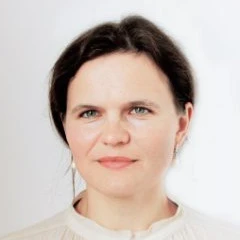
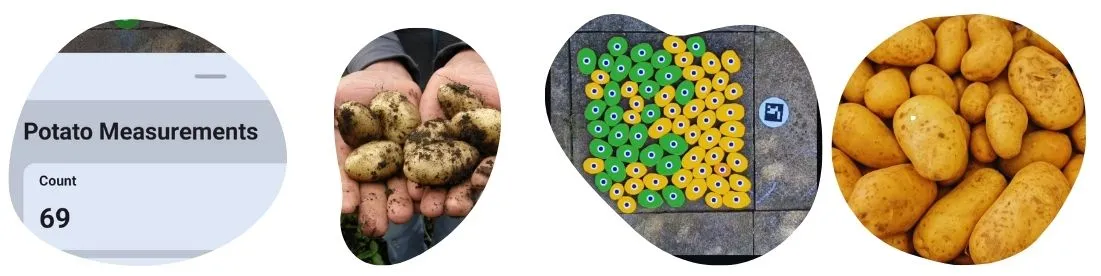
What is the international standard for potato grading?
Potato grading by the USDA (United States Department of Agriculture) is a system for classifying potatoes based on their quality, size, and appearance. This grading system helps ensure consistency in the market and provides consumers and buyers with standardized information about the potatoes they’re purchasing. Here’s a brief overview of the USDA potato grading system:
- U.S. Extra No. 1: The highest grade, these potatoes are the best quality with very few defects.
- U.S. No. 1: Good quality potatoes with minor defects allowed.
- U.S. Commercial: Similar to U.S. No. 1, but with slightly more lenient standards for defects.
- U.S. No. 2: Lower quality potatoes with more defects allowed, often used for processing.
Each grade has specific requirements for factors such as:
- Size
- Shape
- Cleanliness
- Firmness
- Damage (from cuts, bruises, disease, etc.)
- Internal defects
The exact specifications can vary depending on the type of potato (e.g., round white, long white, russet) and whether they’re intended for fresh market or processing.
Mobile application Petiole Pro provides information about potato grading in accordance with USDA requirements
Where to read more about USDA Potato Grading?
Check the official visual aids for potatoes, developed by the USDA.
- Purpose: The visual aids are designed as a reference tool for identifying various potato defects, such as sprout clusters, netting, and elephant hide. They are not intended for official use.
- Updates: The index of official visual aids includes updates from as far back as 1998, with recent additions made in 2017 to assist inspectors with visual standards for different potato conditions.
- Identification: Certain conditions like “well-shaped” potatoes and early stages of elephant hide are listed as “identification only” and are not scorable, indicating that these are for visual guidance rather than grading.
USDA Guide for potato grading. Source: Official Visual Aids for Potato, United States Department of Agriculture
Performance Optimization and Experimental Study of Small-Scale Potato-Grading Device
Country: China 🇨🇳 Published: 24 May 2024
The study aimed to enhance the efficiency of a small-scale potato-grading device by optimizing key operational parameters and validating them through experiments. Researchers employed an orthogonal experimental design, focusing on five main factors (e.g., slide rail height, chain speed). They conducted indoor tests using accuracy and efficiency as indicators to refine the grading device performance. Validation tests confirmed the optimal configurations.
Key findings include a 3.84% improvement in grading accuracy (from 91.04% to 94.88%) and a 12.94% increase in efficiency (from 12.35 t/h to 13.9477 t/h). These advancements signify enhanced productivity and precision in potato sorting. Agricultural machinery developers and small-to-medium-scale potato farmers can practically apply these findings.
Technologies used
- Grading device with MRP unit
- Orthogonal experiment setup
- Conveyor belt system
Main figures of the research
Structural diagram of the MRP-type grading device for potatoes. Source: Zhao et al., 2024
In figure above - MRP, movable rotating plate. 1: Drive sprocket; 2: body frame; 3: the first level potato output device; 4: the second level potato output device; 5: bearing seat; 6: MRP; 7: sprocket shaft; 8: intermediate crossbeam; 9: herringbone baffle; 10: slide rail; 11: chain; 12: The third level potato output device.
Potato-grading principles schematic diagram. Source: Zhao et al., 2024
In the figure above: 1: Sprocket; 2: horizontal slide rail height adjustment device; 3: chain; 4: MRP; 5: horizontal slide rail; 6: inclined slide rail; 7: potato; 8: inclined slide rail angle adjustment device.
First-generation grading device test process. Source: Zhao et al., 2024
Grading unit slide mechanism: 1: fixed crossbeam; 2: horizontal slide rail; 3: horizontal slide rail height adjustment device; 4: inclined slide rail; 5: inclined slide rail angle adjustment device. Source: Zhao et al., 2024
Optimized slide mechanism: 1: fixed crossbeam; 2: horizontal slide rail; 3: horizontal slide rail height adjustment device; 4: first-grade inclined slide rail; 5: inclined slide rail angle adjustment device; 6: second-grade inclined slide rail; 7: slide rail tail rod. Source: Zhao et al., 2024
Bagging device. Source: Zhao et al., 2024
Individual potato weighing: (a) small potato; (b) medium potato; (c) large potato. Source: Zhao et al., 2024
Potatoes after grouping. Source: Zhao et al., 2024
Potato-grading process: 1: conveyor belt; 2: drive chain; 3: sprocket; 4: first-level potato output device; 5: MRP; 6: second-level potato output device; 7: grading device. Source: Zhao et al., 2024
Line graph matching A and B levels in grading efficiency. Source: Zhao et al., 2024
Line graph matching B and C levels in grading efficiency. Source: Zhao et al., 2024
Comparison of the grading performances of similar small- and medium-sized potato-grading devices. Source: Zhao et al., 2024
Other research papers about potato grading (paid access is provided by the publishers)
Tuber Ruler: a mobile application for evaluating image-based potato tuber size
The Tuber Ruler app, developed for Android, uses image analysis and machine learning to measure the length-to-width ratio of potato tubers, offering an efficient alternative to manual methods. It delivers highly accurate results across various potato types and backgrounds, with a correlation coefficient of up to 0.99 and minimal error. The app performs well even with lower image resolutions and natural environments, making it a valuable tool for the potato industry.
This research presents a microscopy image-based vegetable quality assessment system, leveraging deep learning (DL) on edge devices. The prototype uses low-cost Foldscope microscopy with smartphones and a new lightweight DL architecture for on-device analysis, focusing on cell-level attributes like starch content in potatoes. The system achieves rapid and accurate assessments, with a recognition accuracy of 88.8% and an F1-score of 85.83, processing starch content in 24 seconds. This efficient, affordable solution is portable and can extend to other vegetables, supporting real-time nutrient quantification without external servers.
Grading and Defect Detection in Potatoes Using Deep Learning
This paper presents a practical approach for grading potatoes and classifying their defects using deep learning techniques. Inspired by tumor detection in medical images, the method uses U-Net for image segmentation of large potato batches and computer vision algorithms to refine object outlines. Transfer learning is then applied to classify defects like greening, rotting, and sprouting. The approach combines segmentation, distance transformation, and watershed algorithms to deliver accurate potato defect detection, aiming to provide an effective solution for agricultural quality assessment. Mobile application Petiole Pro for potato farming
Mobile application Petiole Pro allows to conduct individual potato phenotyping and measuring with smartphone Mobile application Petiole Pro allows to conduct individual potato phenotyping and measuring with smartphone